Every company of every size — even organizations of just one person — is navigating a data avalanche problem. There’s more instruction on hand than organizations know how to use or conduct.
Every team — from product to marketing and IT to engineering — is generating data. A powerful analytics stack is foundational to making sense of it all. Investing in a robust and effective analytics stack is a demand for a modern business to compete. It empowers each team across the organization to make data-driven decisions, with access to reporting and ad hoc analysis.
What is an Analytics Stack?
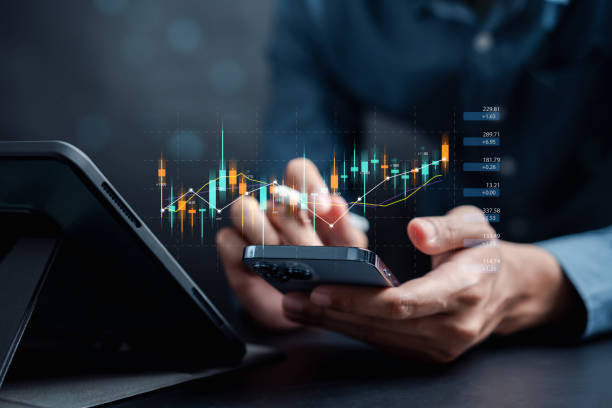
Apply this idea of the “stack” to the weight of data infrastructure maintenance we described above, and it becomes clear that having a well-functioning analytics stack is crucial for any company that wants to cultivate data and extract insights from it. However, as soon as a company’s executives recognize the importance of their data and begin to ask questions about it, they will likely discover that their organization lacks the infrastructure to access their data. Every layer of the analytics stack represents a problem that needs to be solved a skill set is required to solve it.
When to Build an Analytics Stack:
When companies prepare to build a data stack, they usually fall into one of two groups: The first is small organizations that don’t have anything and are starting with a clean slate. The second group includes organizations that have a poorly architected or failing system. Both situations justify an approach.
Usually, organizations have done their strategic planning, and know-how data can help them. Still, they feel that the systems they’re using—usually just the systems they use to run their business—are not powerful enough or don’t provide the level of detail for their analytics practice.
Data Tracking and Collection:
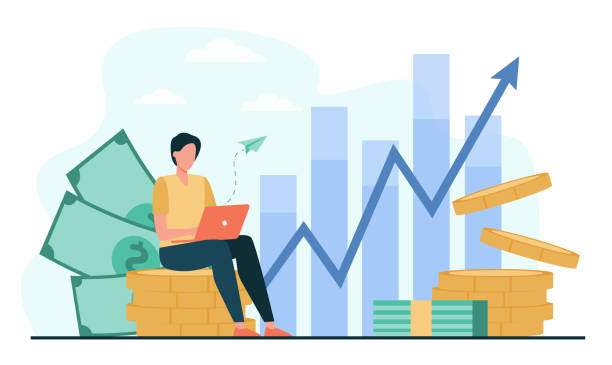
Data tracking and collection is the first milestone in building your analytics stack. Selecting a solution is fundamental to understanding who is doing what on your website or product. Note: It is necessary to remember which downstream platforms or systems you’ll want to send this data to, as each solution has a different selection of integrations.
In the video below, you’ll see a snapshot within Indicative that shows how creating a profile can change how likely a person is toed by making an in-app purchase. This is also referred to as event data.
The data collection step in product and marketing analytics consists of three important components:
1. Data warehouse:
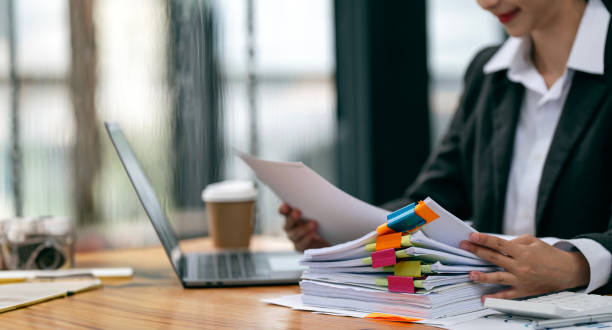
A data warehouse is a centralized storage container that establishes company data accessible to different organizational departments. Out of all of the components of a data analytics stack, data warehouse technology has experienced the most evolution in the last several years.
Here are some factors that you may want to consider when choosing your data warehouse:
- Scalability, so that you can easily store and transform terabytes of data with a few simple processes
- On-demand pricing, meaning that your data warehouse can evolve with your business
- Easy setup so that it’s possible to get up and running quickly
- Solid customer support in case something goes wrong
2. Real-time streaming of user behavior data:
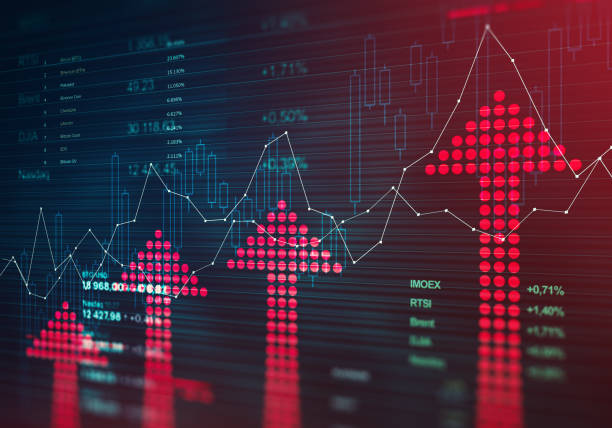
Real-time streams collect user behavior data from your app or website. Tools like Renta script SDK track all the user activity on your website or app and send it to your warehouse. The important thing here is that tools like this support server-side integration and provide data collection as first-party data, bypassing third-party cookie restrictions. It allows for a higher quality of data.
Real-time data streaming tools are good alternatives to Google Analytics 360. They still ensure that you don’t violate the GDPR and that the quality of your data is not adjusted by intelligence tracking preventions while being much more affordable.
3. ETL vs. ELT:
These acronyms correspond to data integration processes for transferring information. With ETL, data is recited, transformed, and then loaded. It is the traditional method for data warehousing.
ELT swaps those last two steps — extracting, loading, and transforming. The difference alters both when and where data transformation is right. Using ETL, transformation happens before loading and is right within the ETL platform. With ELT, data is true to the data warehouse before the transformation. There are two main implications of that distinction:
- Data privacy. For companies troubled with privacy, ETL allows you to clean, mask, and secure data before moving it to the data warehouse.
- Speed. ETL front-loads transformation and back-loads speed, making it faster to pull analysis-ready data from the warehouse. ELT does the opposite — data loads more quickly, but it will take time to transform it before any investigation can be better.
Final thought:
Data is the driver for any modern business. It can give you insights into how your customers interact with your products and how you can make that interaction better for the final user and more profitable for you. To be able to find these insights, you need to set up an effective analytics stack that will gather and process all the information for you.
A modern analytics stack should reflect a simple three-step process: collecting-processing-executing. All these steps can be automated and optimized – and once all that is set, you can proceed to interpreting and using that data to grow your business.